September - November 2021
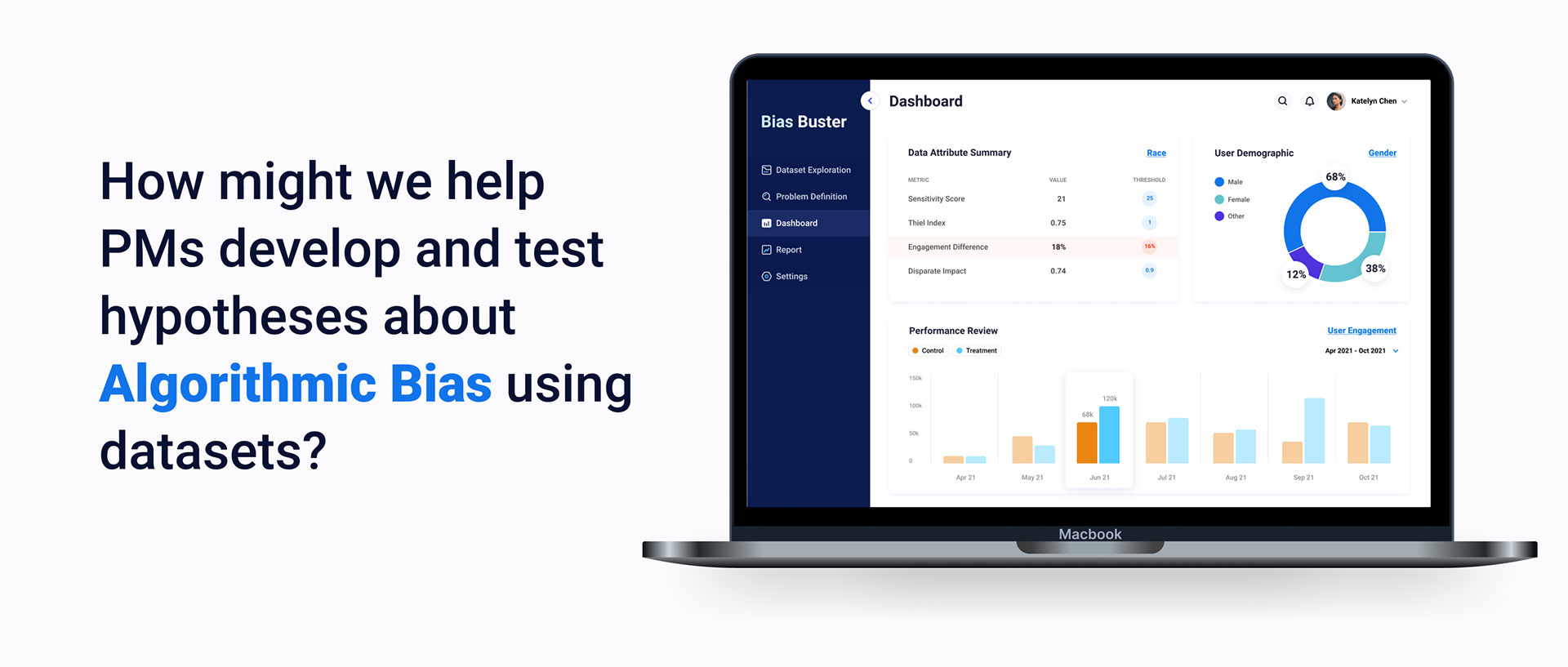
project goal overview
Project Background
Imagine you are a PM at Twitter who has received a data set of users commenting and discussing algorithmic bias on the app. Your job is to take this data and turn it into a project report with actionable tasks for you and your team to address these biases.
What might fairness-related harm look like in the context of this dataset? How can you leverage existing crowdsourcing data on Twitter to properly scope a project and create an actionable report to give to your managers?
My Role & Contributions
Project Manager - kept track of meeting times, agendas, and timelines for milestone deliverables. created documents, organize files, and submit deliverables for the team
Research Lead - developed draft guides for contextual inquiries, interviews, and consent forms.Problem Space
algorithmic bias | Twitter | crowdsourcing user report data | product/project management
After initially speaking with many software engineers and product managers, we found that there isn’t a good system in place to follow for investigating and improving algorithmic bias. They need to be able to provide a summary of the data with metrics that can help identify bias and list the potential scope of the impact of the bias. There is also a need to help the PM provide evidence of the problem and develop a high-level scaffolding problem-finding process.
Methods
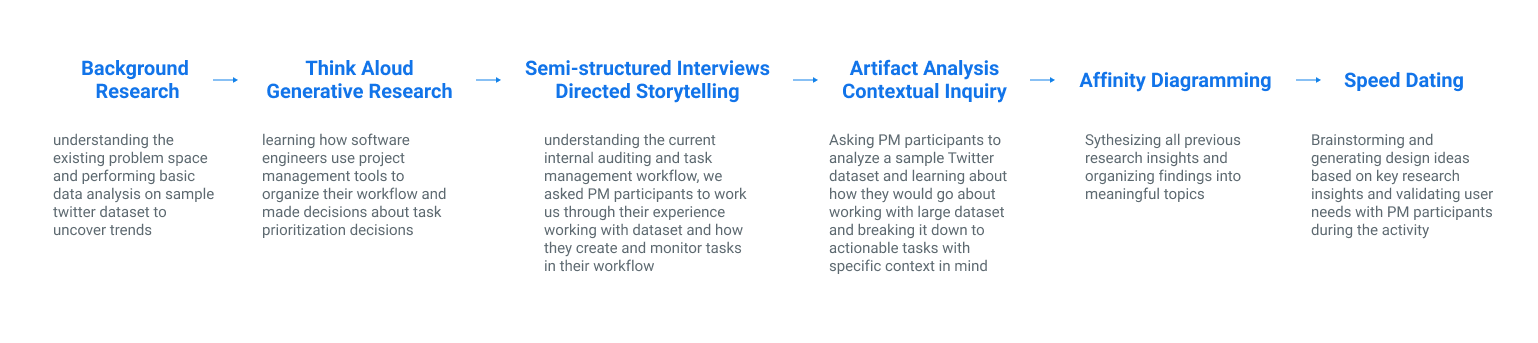
research methods high level overview
To figure out which stakeholders we should target, and their specific needs and pain points, we conducted multiple interviews, such as contextual inquiries, think-aloud protocol, semi-structured interviews, and speed dating. After each set of interviews, we reflected on using different methods including usability findings template, interpretation notes, affinity diagrams, and empathy diagrams, which helped us narrow our focus to product managers and their role when addressing algorithmic bias.
Evidence (User Quotes)
“SWEs are not autonomous as you think”
“Before allocating resources to work on a problem, PMs need to identify what the problem is in the first place”
“PMs don't have the time to look through a raw dataset -- they want it to be broken down by topic, they want it synthesized, they might pull out example quotes”
“PMs often have more problems than they have resources for.”
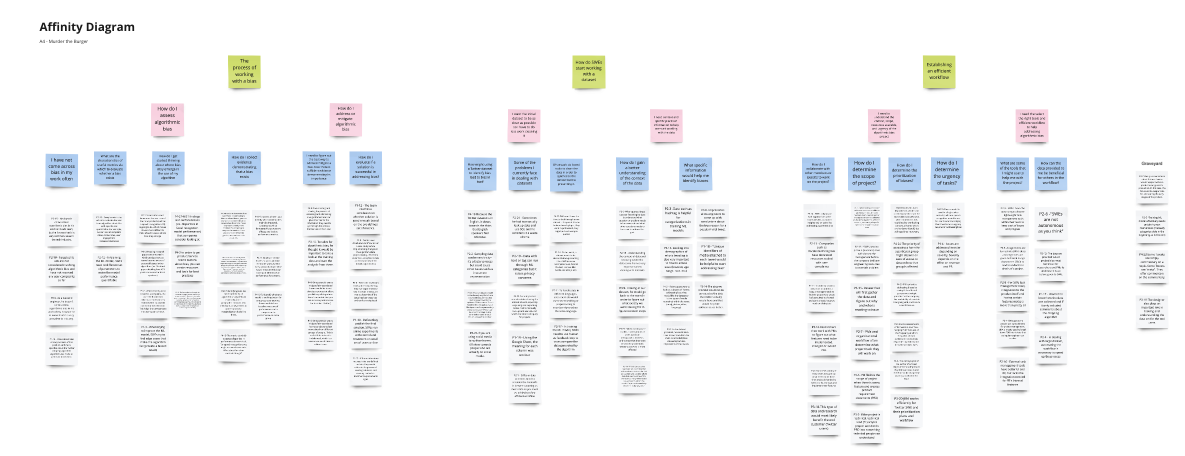
Affinity Diagram using contextual inquiry, artifact analysis interpretation notes
Key Insights
- PMs speak with domain experts before creating the scope and workflow of a project.
- PMs prefer tools that are assistive and not agentive.
- Datasets need to be cleaned and processed before they are useful to software engineers or PMs.
- The context surrounding the data is important for problem-solving -- where the data comes from, what the problem is, and what information is missing.
- Urgency of tasks depends on how high the stakes are (generally based on revenue or PR).
Solution
Our solution aims to ease this transition and help PMs with their problem-solving and project management. Our assistive bias buster tool will allow PMs to explore the dataset by allowing them to reflect on the dataset and help determine if an algorithm is biased. This is meant to serve as a guide that can be customized as they work through the reflection process. The tool also can assist with defining a problem by allowing them to develop hypotheses based on the data collected. They will be able to develop insights and highlight key user quotes and snapshots that back up their hypothesis. The PMs would also be able to get an overview of the data via a data dashboard that would provide the demographics of the user, the data attribute summary, and a performance review of the algorithm. All this information can be used to develop a report that illustrates how to move forward to address the biases identified.
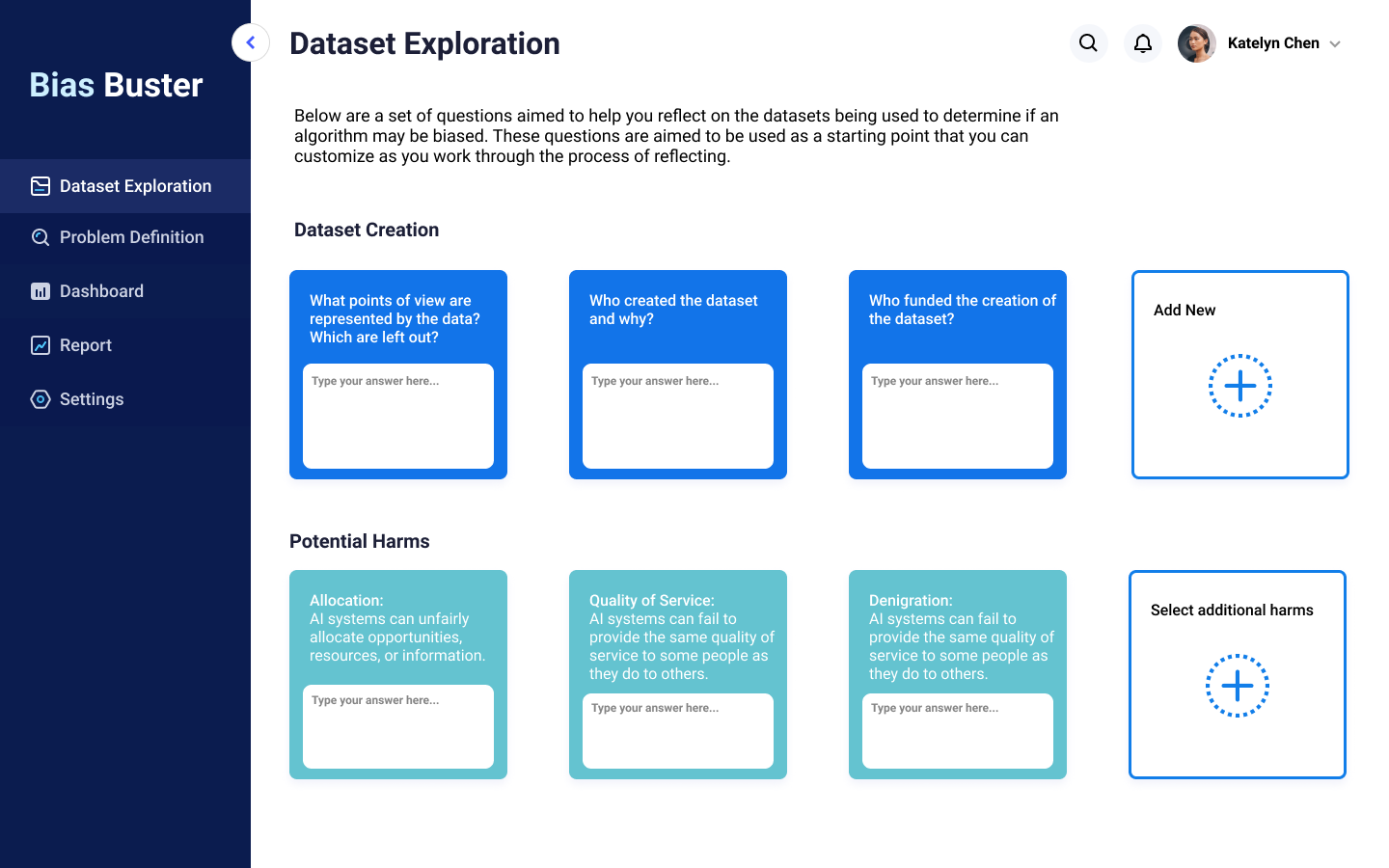
dataset exploration
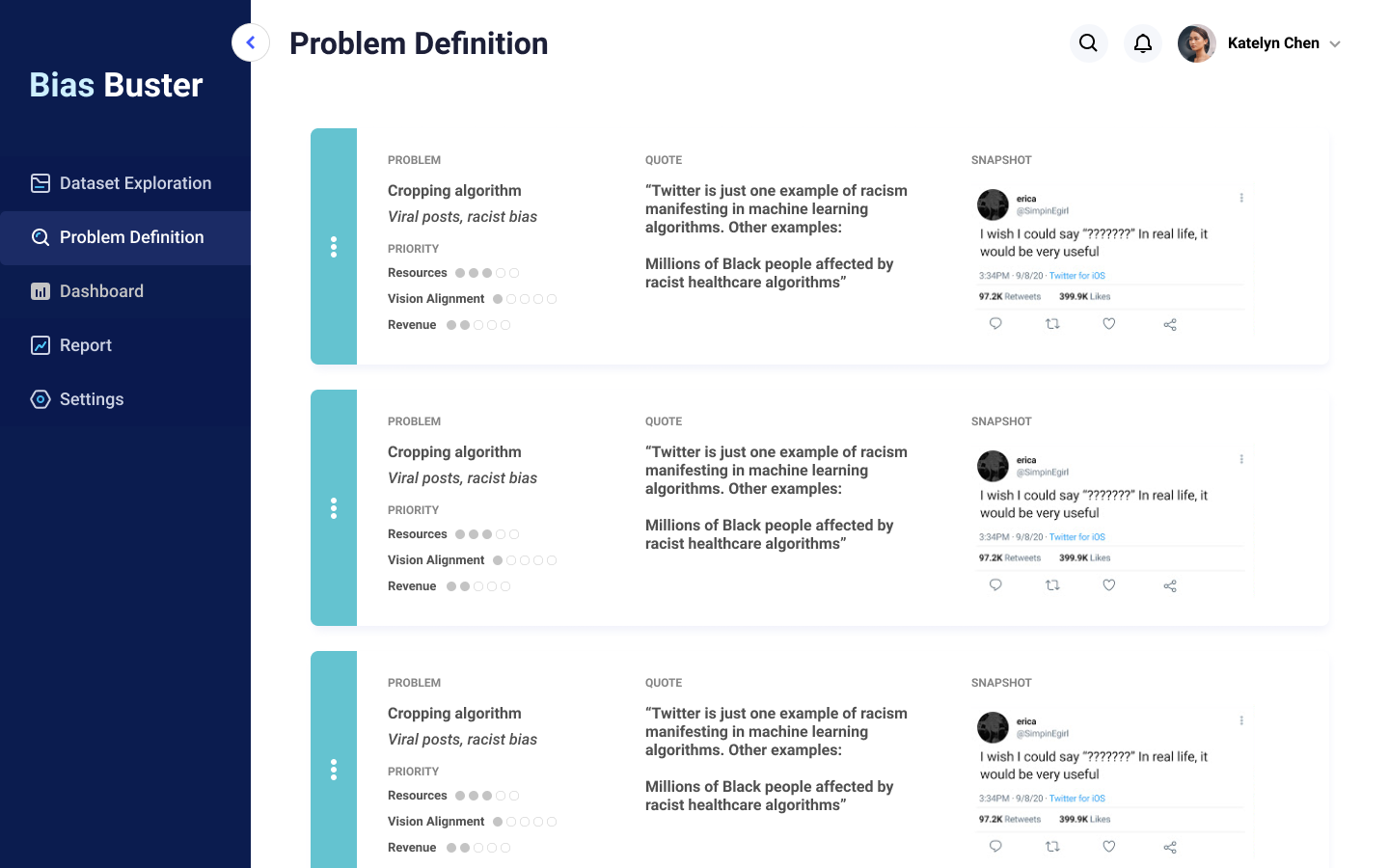
problem definition
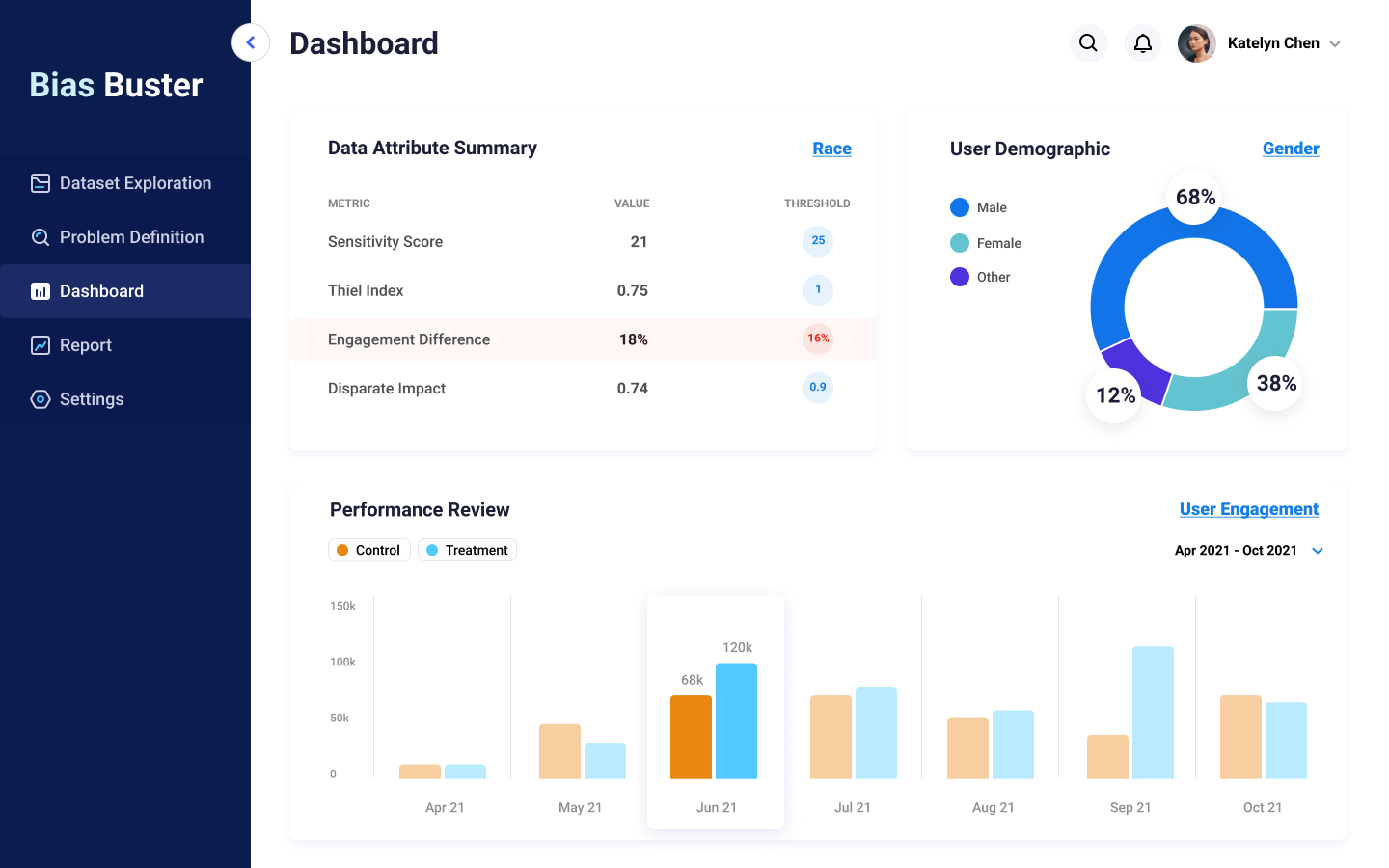
dashboard
Team Members
(best team <3)
Jessica Lin | Ahana Mukhopadhyay | Mabel Tsado